Hey there! RFM (Recency, Frequency, Monetary) analysis is a powerful tool for getting to know your customers better. But like any tool, it can be easy to make mistakes if you're not careful. Let’s chat about some common pitfalls in RFM analysis and how to avoid them with a few best practices.
Common Pitfalls in RFM Analysis
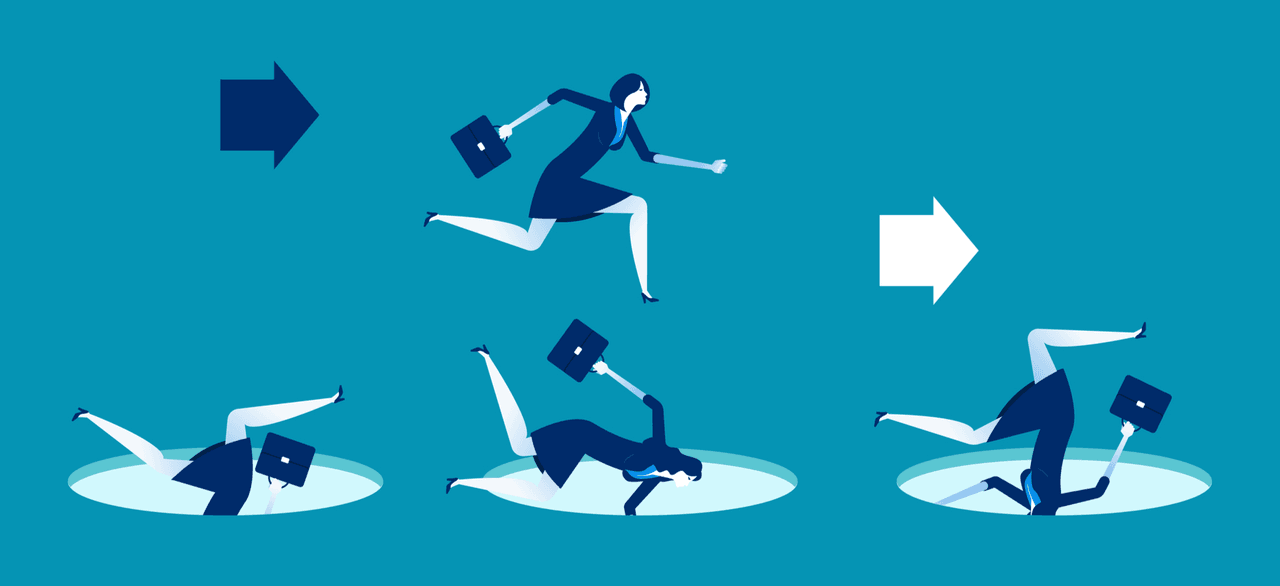
- Relying Only on RFM Scores:
- While RFM scores provide valuable insights, they don't capture the whole picture. It's tempting to see these scores as the ultimate indicator of customer behavior, but customers are more complex than just numbers. To get a comprehensive view, you need to combine RFM scores with other metrics like customer lifetime value (CLV) and qualitative data such as customer feedback. This way, you'll understand not just what your customers are doing, but why they're doing it.
- Ignoring the Context:
- Sometimes, a high recency score can be misleading. For instance, a customer who just bought something during a big sale event might not be a regular shopper. It’s important to consider the broader context when analyzing RFM scores. Look at the data over a longer period and account for seasonal trends or promotional spikes. This will help you avoid overestimating the loyalty of customers who may have only been drawn in by a temporary deal.
- Over-Simplifying Segments:
- Grouping customers into broad categories based on RFM scores alone can lead to missed opportunities. It might seem efficient, but this approach can oversimplify the diverse needs and behaviors of your customers. Instead, try to create more nuanced segments by incorporating additional data points like demographics, purchase preferences, and behavioral patterns. This detailed segmentation allows for more personalized marketing efforts, leading to better engagement and retention.
- Not Updating Scores Regularly:
- Customer behaviors and preferences can change rapidly. If you're not updating your RFM scores regularly, you might be working with outdated information. To keep your data relevant, set a schedule for regular updates. This ensures that your marketing strategies are always based on the most current customer behaviors, allowing you to adapt quickly to any changes.
- Misinterpreting High Monetary Scores:
- High monetary scores are not always indicative of a customer's true value. Sometimes, a customer makes a big purchase only once and never comes back. It's crucial to look at high monetary scores alongside recency and frequency to understand the full picture. A customer who spends moderately but frequently may be more valuable in the long run than someone who spends a lot just once.
Best Practices for Effective RFM Analysis
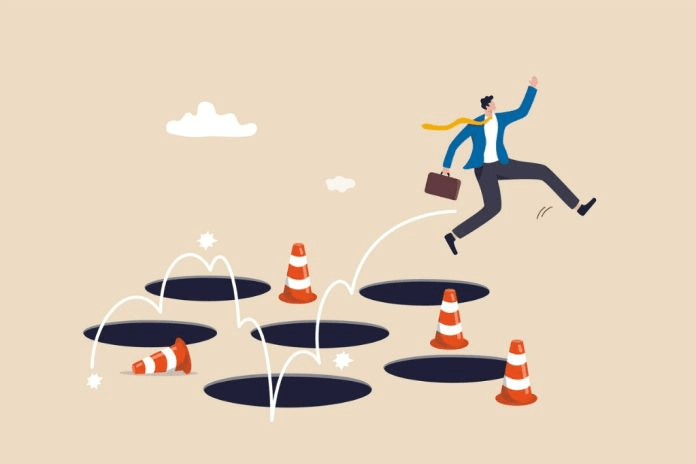
- Integrate RFM with Other Analytical Methods:
- Don’t rely solely on RFM scores. Integrate them with other analyses like CLV and cohort analysis to get a more rounded view of your customers. This combined approach provides deeper insights and helps you make more informed decisions.
- Contextualize Your Findings:
- Always consider the broader context of your sales data. Are there seasonal trends, promotional events, or external factors influencing your customers’ behavior? Understanding the context will help you make more accurate interpretations of your RFM data.
- **Segment with ** Precision :
- Go beyond basic RFM scores to create more detailed customer segments. Use additional data points such as customer demographics, preferences, and feedback to tailor your marketing strategies more precisely. This nuanced approach will lead to better engagement and customer loyalty.
- Regularly Update Your Scores:
- Keep your RFM scores up to date to reflect the latest customer behavior. Regular updates ensure you’re working with accurate data and can adapt your strategies to changing patterns. This proactive approach helps you stay ahead of customer needs and preferences.
- Combine Quantitative and Qualitative Data:
- Use both quantitative data (like RFM scores) and qualitative insights (like customer feedback) to get a fuller understanding of your customers. This holistic approach allows you to address not just what your customers are doing, but why they are doing it, leading to more effective marketing strategies.
- Test and Iterate:
- Continuously test different strategies and iterate based on the results. Use A/B testing to see what works best for different customer segments and refine your approach accordingly. This experimental mindset will help you discover the most effective ways to engage and retain your customers.
Wrapping Up
RFM analysis is a fantastic tool, but it's not without its pitfalls. By staying aware of these common mistakes and following some best practices, you can get the most out of your RFM analysis and better understand your customers.
I hope these tips help you navigate the world of RFM analysis with confidence. Remember, it's all about getting to know your customers better and using that knowledge to serve them better. If you’ve got any questions or want to share your own experiences, I’m all ears. Happy analyzing!